PaletteML enables AI development teams to effectively plan, execute, and manage AI projects through our data-driven project management platform.
Close your eyes and envision yourself in your ideal future. Bask there for a minute. Take in the sights, sounds, and smells of this dream land. And when you’re ready, come back down to Earth.
Now while each person’s utopia is completely different, I’m willing to bet that the first thing that you pictured for yourself was not sitting at a desk for your 9-5 job. This is especially likely if you are a Millennial or Gen Z, of which 51% own or intend to own a business at some point in their lifetime (Inc). Increasingly, younger generations are becoming keen on more flexible work schedules and placing a higher value on their quality of life. All this is to say, your ideal state probably was not set in a corporate office.
Perhaps when I prompted your imagination, you pictured yourself in an exotic destination—maybe the Caribbean islands, Amazon rainforest, or Indian jungle. As it turns out, one of iVenture’s founders, Kartik Hegde, did just the opposite. He left his life in paradise—surrounded by nature, biological diversity, and serenity in rural parts of western India—to pursue a much fiercer ambition.
As a persevering, optimistic, and goal-driven leader, Hegde decided to create his own startup company called PaletteML, a stack of applications aiming to change how machine learning (ML) projects are planned and managed, particularly in small to medium-sized companies. His vision is to build a tool that seamlessly integrates data into organizational tools, which will make the development of this critical technology much more efficient. This is especially timely given that 87% of the ML market consists of early adopters whose technology never makes it to production (D2IQ).
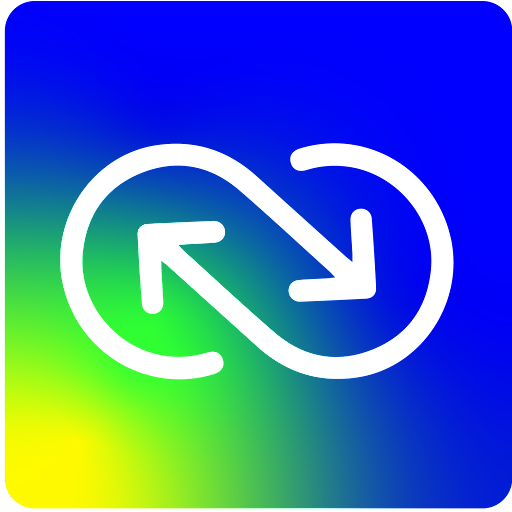
While ML and artificial intelligence (AI) may appear a foreign and dystopic concept to some of us, the application of this technology is extremely pertinent in today’s world. For example, voice recognition on mobile phones, filtering of spam emails, and internet search engines all use machine learning to anthropomorphize the user experience.
Fascinatingly, this competency is something that Hegde learned about at a young age, as his interest in computers was sparked when his uncle gave him his very first laptop. Sure, the machine had a dinosaur-speed Windows 95 operating system and a roller ball instead of a computer mouse, but he loved messing around with the technology and inviting his friends over to probe around the device.
His curiosity lent itself to compassion when he realized just how transformational his interest in technology could be. Growing up on a family farm in rural India, life was not easy. There was a lot of manual labor involved, which was particularly cumbersome during hot days, monsoon season, and the middle of the night. One recurring problem that the family endured was that Hegde’s father had to hike an exceptionally long distance to hit a button to turn on the irrigation system for their crop fields. Knowing how much frustration and exhaustion this caused, Hegde decided to put his computational skills to good use for the benefit of his family.
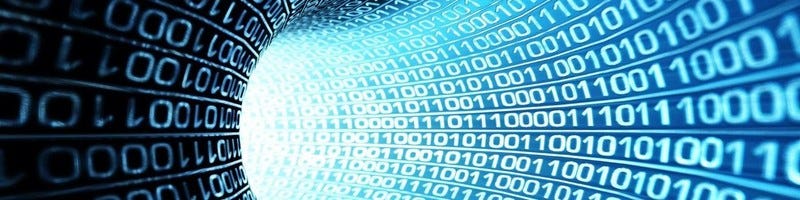
He built a device where he could call a telephone number on his mobile device and it would turn the irrigation system on or off. Hegde had just started learning about coding and engineering as an undergraduate student in India, and he figured out a way to put his schooling to good use—so much so that other farmers started requesting his technology as well. This project was his first run in with the idea that innovation is reared from frustration.
Years down the line, this sentiment transpired into his concept for PaletteML. Hegde is vocal about the fact that, technologically, ML is currently in the stage of the internet in the 1990s. It is currently quite rudimentary but has clear potential to “disrupt almost every field we can think of.” Therefore, Hegde professes,
“It will be in everyone’s interest to unblock machine learning from what it should be.”
One of the main learning blocks to ML progress is that the design of these new functionalities is fundamentally different from traditional software design. If you want to build software, for example, you can build it through engineering and pre-existing models. For machine learning, on the other hand, you don’t know if your idea can even be built. You must experiment and reason inductively to see if your concept is verifiable.
Inherently, the need to proceed in the face of uncertainty leads to poor developer productivity and ultimately slower innovation. In fact, Hegde has interviewed about 50 different ML developers, and nearly all of them expressed frustration in the organization of their process and the productivity of their work. Sympathizing with this struggle, PaletteML seeks to address the rhetorical question,
How can we provide structure and standardization to increase productivity of machine learning development?
Inquiries such as these have always been center frame for Hegde, which led him to pursue his PhD in computer science. Although he attributes his doctoral program to earning a great wealth of knowledge and building relationships with his academic mentor Christopher Fletcher, his ultimate goal throughout all this has been to build technology that could help people. His PhD has taught him to think about problems in new ways, which helped him tremendously on his startup journey.
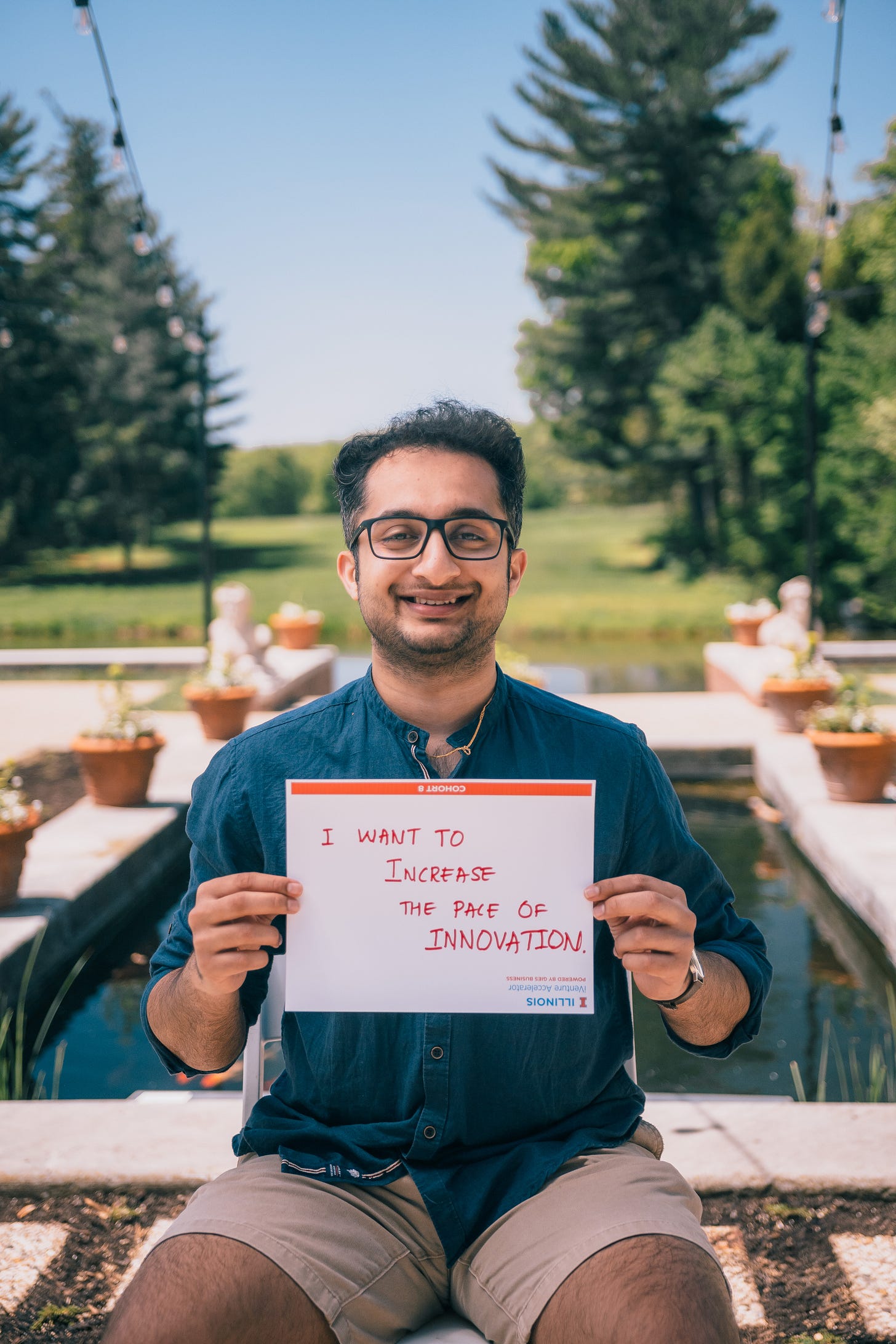
One unexpected component of PaletteML’s experience in iVenture, especially as a software company, has been bridging creativity with technology. As a coder and student of computer science, Hegde has found himself vexed by the computational component of the company. By joining iVenture, however, he has been forced to look at the business model and marketing side of things, which has been an exciting challenge. Given his limited bandwidth, both experientially and time-wise, he has also begun the process of expanding the team and offloading some of the work that he has been diligently working on as a sole fellow in iVenture to a new co-worker.
Most recently, PaletteML has enthusiastically released their minimum viable product (MVP)! The goal with this release is to vet the company’s hypothesis that project management tools are lacking in their ability to link relevant data. If this early-stage software, similar to Trello or Notion for machine learning, receives recognition and positive feedback, then this ecosystem of apps can continue onto its next stage as planned.
Here’s hoping that this unique and vital toolset promoting productivity, innovation, standardization, and empowerment takes off—not only for the sake of PaletteML, but for the sake of untapped machine learning across the globe! If you’re interested in learning more about PaletteML or following Hegde’s journey, check out their website!